📝 Summary
In statistics and probability, a random variable is a core concept that represents numerical outcomes of random phenomena. There are two main types: discrete variables, which have countable outcomes, and continuous variables, which can take any value within a range. Probability distributions describe the likelihood of these outcomes, with discrete distributions like the binomial distribution and continuous ones like the normal distribution. Understanding these topics has vast applications in sectors such as finance, healthcare, and sports analytics, helping quantify uncertainty and make informed decisions.
Understanding Random Variables and Their Probability Distributions
In the world of statistics and probability, we often encounter terms that help us quantify uncertainty. One such term is a random variable. It serves as a fundamental concept in probability theory and is essential for statistical analysis. In this article, we will explore what random variables are, the different types they come in, and how their probability distributions work.
What is a Random Variable?
A random variable is a variable whose possible values are numerical outcomes of a random phenomenon. It is a way to systematically assign numbers to outcomes of random processes. There are two main types of random variables: discrete and continuous.
- Discrete Random Variables: These variables can take on a finite or countably infinite number of values. For example, the number of heads in five coin tosses or the number of cars passing through a toll booth in an hour are discrete random variables. Their outcomes are distinct and can be counted.
- Continuous Random Variables: In contrast, these can take on any value within a given range. For instance, the height of students in a class or the time it takes to run a race are continuous random variables, as they can exist at any point within an interval.
Definition
Finite: This term means something that has an end or limit. In terms of numbers, finite represents countable sets. Countably Infinite: This describes a set that has the same size as the set of natural numbers, meaning it can be listed in a sequence (like 1, 2, 3, …).
Examples
If we roll a dice three times, the outcomes are discrete random variables—the possible values are 1, 2, 3, 4, 5, and 6.
Probability Distributions
A probability distribution provides a function that describes the likelihood of different outcomes of a random variable. There are different types of probability distributions corresponding to discrete and continuous random variables. Understanding these distributions helps us determine how likely different outcomes are.
- Discrete Probability Distribution: In this distribution, the function assigns probabilities to each possible outcome. The probabilities must sum to 1. An example is the binomial distribution, which gives the probability of having a fixed number of successes in a fixed number of Bernoulli trials.
- Continuous Probability Distribution: Here, the probability is described using a function called the probability density function (PDF). One common example is the normal distribution, which is a bell-shaped curve that is symmetric about the mean.
Definition
Bernoulli Trials: These are random experiments where there are only two possible outcomes: success or failure. Probability Density Function (PDF): This function describes the likelihood of a continuous random variable taking on certain values.
Examples
An example of a discrete probability distribution is flipping a coin. The probability of obtaining heads is 0.5 and for tails, it is also 0.5.
Key Characteristics of Probability Distributions
Understanding the key characteristics of probability distributions can enhance our comprehension of random variables. Here are some important features:
- Mean (Expected Value): This is the average value of all possible outcomes, weighted by their probabilities. It gives us the central tendency of the distribution.
- Variance: This measures the spread of the random variable around the mean. A higher variance indicates that the values are more spread out.
- Standard Deviation: The square root of variance, it provides another measure of the spread of a distribution. A low standard deviation means that the values cluster closely around the mean.
Discrete Probability Distributions Explained
Focusing on discrete distributions, two popular types are the binomial distribution and the Poisson distribution. The binomial distribution is useful for situations involving two possible outcomes. For example, consider a student answering a series of multiple-choice questions—determining how many they answered correctly follows a binomial distribution.
Examples
If a student guesses the answer to 5 multiple-choice questions, each with 4 options, the number of questions they get right can be modeled using the binomial distribution.
The Poisson distribution, on the other hand, applies to scenarios where we measure the number of events happening within a fixed interval of time or space. An example is counting the number of emails received in an hour or the number of phone calls to a call center in a day.
Definition
Cumulative Distribution Function (CDF): This function gives the probability that a random variable is less than or equal to a certain value.
Continuous Probability Distributions Explained
As we explore continuous distributions, the normal distribution is one of the most significant. It is often referred to as a Gaussian distribution. The majority of values fall near the mean, with probabilities gradually decreasing as you move away from the mean‚’ peak. This distribution is essential due to the Central Limit Theorem, which states that the sum of a large number of independent, identically distributed variables will be approximately normally distributed.
❓Did You Know?
Did you know that the normal distribution is often referred to as the bell curve due to its shape? It is prevalent in real-life situations like heights, test scores, and measurement errors!
Other continuous distributions include the exponential distribution, often used to model the time until an event occurs, such as the time until a radioactive particle decays.
Applications of Random Variables and Probability Distributions
Random variables and their probability distributions have numerous applications across diverse fields. These applications include:
- Finance: In finance, understanding risk and returns relies on probability distributions. Portfolio managers utilize these concepts to evaluate investment options.
- Sports: Athletes and coaches often analyze performance using probability distributions to statistically determine the chances of winning an event.
- Healthcare: Medical researchers use these concepts to ascertain treatment effectiveness or predict patient outcomes based on historical data.
Conclusion
In summary, we have explored the intriguing world of random variables and their probability distributions. These concepts serve as the backbone of statistics and help us quantify uncertainty. Whether we are dealing with discrete or continuous outcomes, understanding the key characteristics enables us to draw insights from data. The applications of these principles are vast and serve essential roles in fields like finance, healthcare, and even sports analytics. By grasping these fundamental ideas, we equip ourselves to tackle real-world problems armed with statistical knowledge.
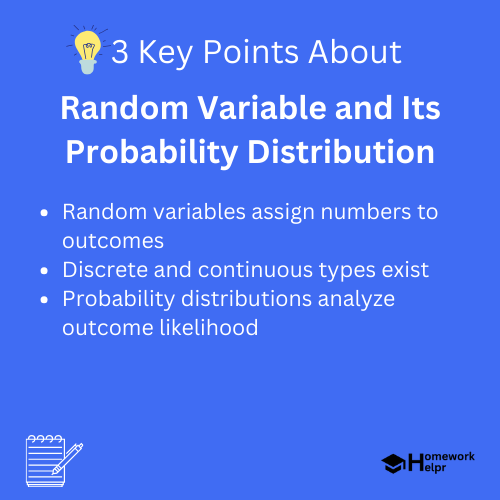
Related Questions on Random Variable and Its Probability Distribution
What are random variables?
Answer: Random variables assign numbers to random outcomes.
What do probability distributions describe?
Answer: They describe the likelihood of random variable outcomes.
What are the main types of probability distributions?
Answer: They are discrete and continuous distributions.
How are random variables used in healthcare?
Answer: They predict patient outcomes based on historical data.