📝 Summary
In the information age, understanding data is crucial. This article addresses three key concepts: Raw Data, Classification of Data, and Variables. Raw data is unprocessed information from various sources, vital for analysis. Data classification includes qualitative (descriptive) and quantitative (numerical) data, each requiring different analytical methods. Variables are any measurable characteristics affecting research outcomes and include independent, dependent, and controlled types. Gaining these skills is essential for navigating an increasingly data-driven world.
Understanding Raw Data, Classification of Data, and Variables
In the age of information, understanding data is vital. Data is all around us, influencing our decisions, businesses, and everyday activities. This article will explore three essential concepts: Raw Data, Classification of Data, and Variables. By the end, you will have a clearer understanding of how data is gathered, categorized, and utilized.
What is Raw Data?
Raw data refers to unprocessed information collected directly from sources without any manipulation or analysis. This data is in its original form, therefore, it is not influenced by any computations or alterations. Raw data can originate from various sources, such as surveys, experiments, and observations. It is essential for further analysis and processing.
Examples of raw data include:
- Responses from survey participants
- Measurements collected from an experiment
- Temperature readings taken over days without adjustments
When working with raw data, researchers must manage significant amounts of information. It can be messy and may contain inaccuracies. However, raw data is important as it allows researchers to maintain the integrity of their findings by not introducing biases through preprocessing.
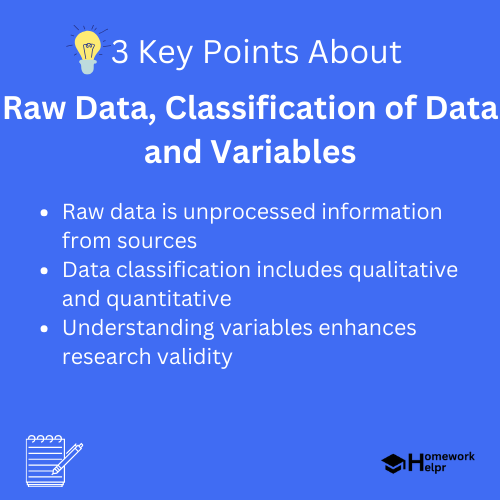
Definition
Classification of Data
Once data is gathered, it must be classified or organized into meaningful categories. This process of classification is crucial for better understanding, interpretation, and analysis of the data. Classifying data can lead to more efficient analysis and provide insights into the information collected.
Data can be classified into various types, primarily: qualitative and quantitative data.
- Qualitative Data: This type of data describes characteristics and can be observed but not measured. For example, colors, opinions, or types of animals fall under qualitative data. It is often collected through interviews or open-ended questions.
- Quantitative Data: This data is numerical and can be measured and compared. Examples include height, weight, or temperature. Quantitative data can further be classified into discrete (countable values) and continuous (measurable values).
Understanding the different types of data helps researchers apply the appropriate statistical methods for analysis. For instance, while qualitative data could be analyzed through thematic analysis, quantitative data could be examined using statistical techniques like mean, median, or mode.
Definition
Qualitative Data: Describes characteristics that can be observed but not measured. Quantitative Data: Consists of numerical information that can be measured and compared.
Understanding Variables
In the realm of statistics and research, variables play a crucial role. A variable is any characteristic, number, or quantity that can be measured or counted. Variables are fundamental because they help researchers understand the relationship between different components in a given experiment or study.
Variables can be classified into different types:
- Independent Variables: These are the variables that are manipulated or changed in an experiment. For example, in a study to determine the effect of study hours on exam scores, the number of study hours would be the independent variable.
- Dependent Variables: These are the outcomes that are measured in response to changes in the independent variable. In our previous example, the exam scores would be the dependent variable.
- Controlled Variables: These are the factors that are kept constant in an experiment to ensure that the results are valid. Examples could be the type of study material or the testing environment.
Understanding these variables allows researchers to draw conclusions and formulate theories based on their findings. Proper identification of variables helps enhance the validity and reliability of the research.
Definition
Independent Variable: A variable that is manipulated or changed in an experiment. Dependent Variable: The outcome that is measured in response to changes in the independent variable. strong>Controlled Variable: The factors kept constant in an experiment to ensure valid results.
Fun Fact About Data
❓Did You Know?
Did you know that the first computer programmer, Ada Lovelace, worked on data algorithms in the 1800s? She is often credited with anticipating the capabilities of modern computing.
Conclusion
Data is a powerful tool that shapes our understanding of the world. From raw data to classification and variables, each aspect plays a significant role in the processing and analysis of information. By grasping these concepts, students and researchers can make better sense of data and apply their findings more effectively.
As you continue your studies, remember that mastering the identification, classification, and manipulation of data will be essential skills in an increasingly data-driven world. Keep exploring, questioning, and learning!
Related Questions on Raw Data, Classification of Data and Variables
What is raw data?
Answer: Raw data is unprocessed information from sources.
Why is data classification important?
Answer: Data classification aids in understanding and analysis.
What are independent variables?
Answer: Independent variables are manipulated in experiments.
How are qualitative and quantitative data different?
Answer: Qualitative data is descriptive, while quantitative is numerical.