📝 Summary
In statistics, sampling is essential for gathering data, but sampling errors can undermine research results. Errors occur when the sample does not accurately represent the population, which may mislead decision-making. Organizations like the NSSO employ methods like stratified and systematic sampling, along with rigorous training, to minimize these errors. Despite their efforts, significant implications arise from sampling errors, including misleading conclusions and a loss of credibility. Thus, understanding and addressing sampling errors is critical for obtaining reliable data for effective policymaking.
Sampling Errors and NSSO
In the world of statistics, sampling plays a crucial role in gathering data for research and analysis. One of the primary challenges researchers face is understanding and minimizing sampling errors. This article will take a deep dive into what sampling errors are, their implications, and how organizations like the National Sample Survey Organization (NSSO) work to mitigate these errors. Let’s explore these concepts in detail.
What is Sampling?
Sampling is the process of selecting a subset of individuals, items, or data points from a larger population to make inferences about that population. This method is widely used in various fields such as social sciences, marketing, and public health. The goal is to understand trends, behaviors, and attitudes without surveying the whole population, which would be time-consuming and expensive.
There are various types of sampling methods, a few of which include:
- Simple Random Sampling: Every member of the population has an equal chance of being selected.
- Stratified Sampling: The population is divided into subgroups, and samples are drawn from each group.
- Systematic Sampling: Items are selected using a fixed interval from a randomly chosen starting point.
Definition
Sampling: The act of selecting a subset of data from a larger set. Stratified Sampling: A method of sampling that involves dividing the population into smaller groups and then taking a sample from each group.
Examples
For instance, if you wanted to conduct a survey about student satisfaction in a school, you might select a group of students from each grade rather than polling every student.
Understanding Sampling Errors
Sampling errors occur when the sample selected does not accurately represent the entire population. This discrepancy between the statistic derived from the sample and the actual population parameter is known as sampling error. It’s important to note that sampling errors can arise from various factors, including:
- Sample Size: Smaller samples are more likely to have higher sampling errors.
- Selection Bias: If certain members of the population have a higher chance of being selected, it could lead to bias.
- Non-Response Bias: If individuals chosen for the sample do not respond, it can skew results.
Understanding sampling errors is crucial since they can lead to incorrect conclusions and decisions based on the data analysis. For example, if the NSSO reports that 70% of people favor a certain policy, but the sample did not represent the demographics accurately, that information might mislead policymakers.
Definition
Selection Bias: A form of bias arising when individuals selected for a sample differ from the population in significant ways. Non-Response Bias: A bias introduced when participants chosen for a survey do not respond.
Examples
If you surveyed only students who use the school library to understand reading habits, your findings might not reflect the entire student population’s reading preferences.
The Role of NSSO
The National Sample Survey Organization (NSSO) is an essential Indian government agency responsible for conducting surveys on various socio-economic parameters. Established in 1950, its primary purpose is to provide reliable data to the Indian government for policy formulation and decision-making. NSSO designs its surveys carefully to minimize sampling errors and ensure representative data.
The {NSSO tackles sampling errors through:
- Methodical Sampling Techniques: They use different sampling methods such as stratified and systematic sampling to ensure that the sample is representative.
- Periodic Updates: The NSSO frequently updates its sampling methodologies and equipment based on the latest statistical techniques.
- Comprehensive Training: NSSO provides rigorous training to its field personnel to minimize biases during data collection.
❓Did You Know?
Did you know that the NSSO conducts large-scale surveys every five years to gather data on household consumption patterns in India? This helps understand economic trends and develop better policies!
Implications of Sampling Errors
The implications of sampling errors can be significant, especially in big organizations like NSSO, where data quality directly affects policy decisions. Sampling errors can lead to:
- Misleading Conclusions: Incorrect sample representation may lead to policies that do not address the real issues faced by the population.
- Financial Consequences: Wrong policies result in inefficient allocation of resources, causing a waste of public funds.
- Loss of Credibility: Continuous reporting of erroneous data can lead to a loss of trust in statistical agencies.
Definition
Allocation of Resources: The distribution of resources based on specific parameters or needs. Credibility: The quality of being trusted and believed in.
Examples
For instance, if a survey concludes that most households have access to clean drinking water based on a biased sample, funds may be directed to unnecessary infrastructural improvements rather than areas that genuinely need them.
Ways to Minimize Sampling Errors
To ensure accurate representations, researchers and organizations like NSSO can implement various strategies to minimize sampling errors. Some effective methods include:
- Increasing Sample Size: A larger sample size generally improves the reliability of results.
- Random Selection: Randomly selecting participants reduces the chance of biases affecting the sample.
- Pre-testing Surveys: Conducting small-scale tests before the main survey helps identify potential issues in sampling methods.
By adopting these strategies, researchers can improve the validity of their conclusions and avoid the pitfalls of sampling errors. For NSSO, maintaining high standards in data collection is paramount for supporting governmental decisions.
Conclusion
Sampling errors present significant challenges in the field of statistics and data collection. Understanding these errors and their implications is vital for researchers, policymakers, and organizations like the NSSO, which strive to provide accurate and representative data. By utilizing appropriate sampling techniques and implementing rigorous data collection protocols, we can reduce sampling errors and ensure that critical decisions are made based on reliable information. As statistics continue to play an essential role in shaping our society, awareness and education on sampling errors remain more critical than ever.
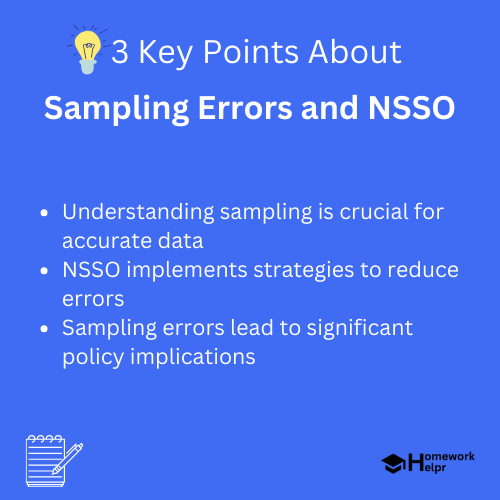
Related Questions on Sampling Errors and NSSO
What is sampling?
Answer: Sampling is selecting a subset from a population.
Why are sampling errors important?
Answer: They can mislead conclusions and decisions.
How does NSSO minimize sampling errors?
Answer: By using rigorous methods and training.
What are the implications of sampling errors?
Answer: They can cause misleading policies and resource misallocation.