📝 Summary
Statistics is a vital field concerning the collection, analysis, and interpretation of data. The Law of Statistics emphasizes that statistical methods must reflect the reality accurately, distinguishing between quantitative and qualitative data. However, a prevalent distrust of statistics exists, often due to misleading presentations, selection bias, and over-extrapolation. These issues can severely affect decision-making, especially in public health and economic policies. With the increase in media and technology, misinterpretations of statistics have become more widespread. Improving statistical literacy is crucial for individuals to critically evaluate data and combat misconceptions, enabling informed decision-making and understanding of statistics’ importance.
Law of Statistics and Distrust of Statistics
Statistics is an essential field that deals with the collection, analysis, interpretation, presentation, and organization of data. As students, you may have heard the saying, “There are three types of lies: lies, damned lies, and statistics.” This phrase underscores the importance of understanding how statistics can be manipulated. Through this article, we will dive into the Law of Statistics and explore why many individuals develop a distrust of statistics, understanding both concepts in detail.
Understanding the Law of Statistics
The Law of Statistics refers to the fundamental principles and guidelines that govern the collection and interpretation of data. One basic principle is that statistics should accurately reflect the reality they are meant to represent. It is crucial that when analyzing data, the methods used are reliable and consistent.
One important aspect of the Law of Statistics is the distinction between different types of data. Statistics can be categorized as:
- Quantitative Data: Numerical data that can be measured. Examples include height, weight, or temperature.
- Qualitative Data: Descriptive data that is categorized based on traits and characteristics. Examples include colors, preferences, or names.
The correct understanding and application of these categories are vital because they affect how we interpret statistics. For instance, quantifying data related to a group‚’ average age and classifying it, enhances meaningful insights.
Definition
Quantitative Data: Data that can be quantified and is subject to arithmetical manipulation.
Qualitative Data: Non-numerical data that mirrors characteristics, attributes, and quality.
Example
If you measured the height of every student in your class, the average height would be an example of quantitative data. If you asked the class to list their favorite ice cream flavors, that would be qualitative data.
Statistics in Real Life
Statistics plays a vital role in various fields like health, economics, sports, and social sciences. Here are some examples:
- In health, statistics help track the spread of diseases and the effectiveness of vaccines.
- In economics, statistics are used to analyze the financial performance of businesses.
- In sports, statistics provide insights into player performance and team strategies.
However, the interpretation of statistics often leads to misunderstandings. For instance, a study may provide baseball batting averages that illustrate one player’s performance compared to another‚’, yet such statistics can be misleading without context.
The Distrust of Statistics
Despite the vital role statistics play, many people harbor a distrust of them. This skepticism often arises from multiple factors:
- Misleading Presentation: Statistics can be presented in a way that distorts the actual truth. Charts and graphs can be manipulated to emphasize specific outcomes while downplaying others.
- Selection Bias: Sometimes, the data selected for analysis may not be representative of the whole population. This can create an inaccurate picture of reality.
- Over-extrapolation: Conclusions based on limited data may lead to incorrect generalizations. This is common in studies where results are sensationalized for media coverage.
Each of these factors can lead to severe implications. When data is misinterpreted, it can result in poor decision-making, affecting various areas from public health policies to economic forecasts.
Definition
Selection Bias: A bias that occurs when the sample is not representative of the population being studied.
Over-extrapolation: Making assumptions about a larger group based on limited data.
Example
If a survey about a new product is only conducted among users who already like that product, the feedback may be overwhelmingly positive, leading to an over-extrapolation regarding its overall popularity.
The Role of Media and Technology
With the rise of technology and social media, the dissemination of statistics has become incredibly rapid. However, it has also led to the manipulation of information in the following ways:
- Incidents of data misrepresentation often circulate virally across platforms, reaching extensive audiences before corrections are made.
- The reliance on clickbait headlines can skew the interpretation of statistical findings. Readers encounter simplifications that may leave out crucial information.
- There’s often a lack of critical analysis among the general public regarding how statistics are derived and presented.
It’s essential to approach statistics critically, questioning the sources and assessing the reliability of data. Always consider the motives behind data presentation, especially from media outlets.
💡Did You Know?
Did you know that a famous study once indicated the correlation between ice cream sales and drowning incidents? However, the link had no causation-it’s summer heat leading to more people buying ice cream and swimming! This illustrates how correlations in statistics do not imply causation.
Improving Statistical Literacy
To combat the distrust associated with statistics, enhancing our statistical literacy is vital. Here‚’ what can be done:
- Engage in educational programs that teach basic statistical principles.
- Learn how to interpret graphs and tables meaningfully.
- Critically evaluate sources of information and data before accepting them as truth.
Improving our understanding of statistics empowers individuals to question misleading presentations and recognize the underlying truths. This fosters a more informed society capable of making sound decisions based on credible data.
Conclusion
The Law of Statistics serves as a guiding framework for determining how data should be handled and analyzed. While statistics provide valuable insights in various fields, the distrust that many people feel towards them stems from their misuse and misinterpretation. By enhancing our statistical literacy and critical understanding, we can navigate the complex world of data with greater assurance, making informed decisions and cherishing the knowledge statistics bring.
Statistics is ultimately a powerful tool, and with the right intent and methods, it can lead to profound insights into our world.
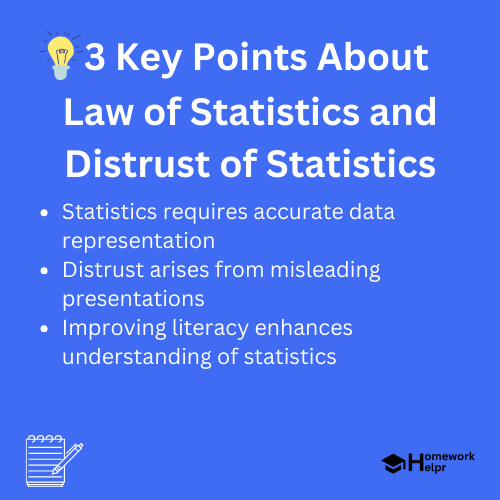
Related Questions on Law of Statistics and Distrust of Statistics
What is the Law of Statistics?
Answer: The Law of Statistics refers to the fundamental principles and guidelines that govern the collection and interpretation of data, ensuring accurate representation of reality.
Why do people distrust statistics?
Answer: People distrust statistics due to factors such as misleading presentations, selection bias, and over-extrapolation, leading to misinterpretations and poor decisions.
How can we improve our statistical literacy?
Answer: Improving statistical literacy can be achieved through educational programs, learning to interpret data accurately, and critically evaluating information sources.
What are the two main types of data in statistics?
Answer: The two main types of data in statistics are quantitative data, which is numerical and measurable, and qualitative data, which is descriptive and categorical.