📝 Summary
Factor analysis is a statistical method used across fields like psychology, finance, and marketing to uncover underlying relationships among variables. This technique helps in dimensionality reduction, simplifying complex datasets into manageable factors without significant information loss. Important steps in factor analysis include data collection, examining the correlation matrix, factor extraction, and interpreting the factors based on contributing variables. Its applications span from identifying latent variables in psychology to understanding consumer behavior in market research. Despite its limitations, such as subjectivity in interpretation and concerns regarding sample size, factor analysis remains essential for drawing meaningful insights from data.
Understanding Factor Analysis
Factor analysis is a powerful statistical method used in various fields such as psychology, finance, and marketing. It helps researchers uncover underlying relationships among a set of variables. By identifying factors, researchers can simplify their data and make sense of complex datasets. In this article, we will explore what factor analysis is, its importance, and how it is performed.
What is Factor Analysis?
At its core, factor analysis is a technique used to reduce data dimensionality. This means condensing a large number of variables into fewer, manageable factors without losing too much information. By doing so, researchers can identify patterns and correlations that may not be immediately visible in the original dataset. In simpler terms, it helps to find the hidden structure in a matrix of data.
Definition
Dimensionality Reduction: A process where the number of variables under consideration is reduced to a smaller number of variables, maintaining the essential information.
Importance of Factor Analysis
Factor analysis plays a crucial role in research for several reasons:
- It simplifies complex data sets, making them easier to analyze.
- It helps to identify latent variables that are not directly observable but influence other variables.
- It improves the accuracy of statistical analyses and models by reducing the noise in large datasets.
- It aids in data interpretation, allowing researchers to draw meaningful conclusions.
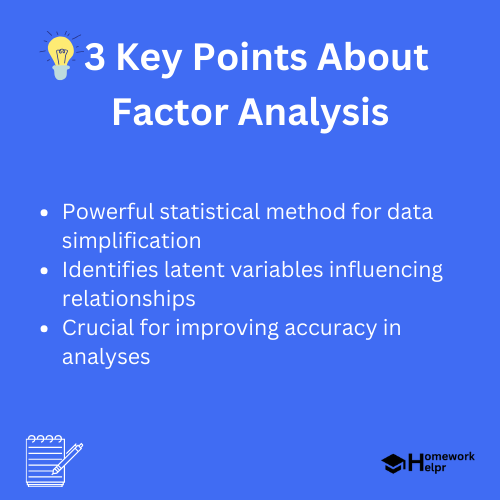
How is Factor Analysis Conducted?
The process of conducting factor analysis involves several key steps:
- Data Collection: Gather data from multiple variables that you want to analyze.
- Correlation Matrix: Examine the correlation coefficients between the variables to determine their relationships.
- Factor Extraction: Use techniques such as Principal Component Analysis (PCA) to extract factors from the data.
- Factor Rotation: Rotate the factors to achieve a clearer and more interpretable structure.
- Interpreting Factors: Analyze and label the factors based on the contributing variables.
Definition
Latent Variables: Variables that are not directly observed or measured but can be inferred from other variables.
Applications of Factor Analysis
Factor analysis is widely applicable across multiple disciplines:
- Psychology: Used in psychometric testing to identify underlying personality traits.
- Finance: Helps in portfolio management by identifying common factors affecting asset returns.
- Market Research: Assists in understanding consumer behavior and preferences by analyzing survey data.
Example
For instance, in a survey about consumer preferences, factor analysis might reveal a common factor of ‘health consciousness’ that influences diverse variables like food choice, brand selection, and shopping behavior.
Understanding Factor Loading
Another essential component of factor analysis is factor loading, which indicates the strength of the relationship between a variable and a factor. Factor loadings range from -1 to 1, where:
- Values close to 1 indicate a strong positive correlation.
- Values close to -1 suggest a strong negative correlation.
- Values near 0 imply little or no correlation.
Definition
Factor Loading: A metric that represents how much a variable is influenced by a particular factor.
Limitations of Factor Analysis
While factor analysis is a useful tool, it has some limitations that researchers must consider:
- Subjectivity in Interpretation: Deciding how to name or interpret factors can be subjective.
- Assumptions: Factor analysis relies on several assumptions, including linearity and normality of data.
- Sample Size: A small sample size can lead to unreliable results and misleading conclusions.
Example of Factor Analysis in Action
Let‚’ take a look at a hypothetical example of factor analysis in action:
- A researcher collects survey data from 300 respondents about their fitness habits, including variables like exercise frequency, diet adherence, and sleep quality.
- After performing factor analysis, the researcher might identify two main factors: ‘Lifestyle’ (combining exercise and diet) and ‘Recovery’ (sleep quality).
- These factors can help the researcher focus on specific interventions targeting lifestyle changes for better health outcomes.
💡Did You Know?
Did you know that factor analysis was first introduced in the 1900s by psychologist Charles Spearman? He used it to explore intelligence testing!
Conclusion
Factor analysis is a valuable method that helps researchers and analysts summarize complex data into interpretable factors. By understanding the core components that influence various variables, we can generate insights which can lead to improved decision-making in numerous fields. Despite its limitations, factor analysis remains an essential tool for enhancing data analysis and research in the modern world. Whether you are a budding psychologist, financial analyst, or market researcher, mastering factor analysis can greatly enrich your statistical toolkit.
Related Questions on Factor Analysis
What is factor analysis?
Answer: Factor analysis is a statistical technique used to reduce data dimensionality by identifying hidden relationships among variables.
What are latent variables?
Answer: Latent variables are those that are not directly measurable but can be inferred from other observed variables.
How does factor analysis simplify data interpretation?
Answer: By condensing a large number of variables into fewer factors, factor analysis reveals patterns and correlations that might not be apparent in raw data.
What is factor loading?
Answer: Factor loading indicates the strength of the relationship between a variable and a factor, ranging from -1 to 1.